Search the repository
Recently published
113208 results
- Jakson Catena DataRaw data of the MS "Mineralogical control and landscape influence on chromium and nickel distribution and bioavailability in tropical ultramafic soils"
- Dataset
- Topology optimization, fabrication, and precision evaluation of automotive flange fork fabricated through laser powder-bed fusion additive manufacturing processThis data contains the nTop file following which, a topology optimization of an automotive flange fork can be performed.
- Dataset
- Fabric DefectA custom dataset of fabric defects was constructed, utilizing images captured from garments with various imperfections. The dataset comprises 2468 high-quality images and each image to ensure quality. The dataset contains images of six types of fabric defects. The dataset has Button-hike (307 images), Broken-button (545 images), Hole (556 images), Color-defect (384 images), Foreign-yarn(334 images), and Sewing-error (317 images). To address potential limitations arising from a finite dataset size, data augmentation techniques were employed. This augmentation process enhanced the model's ability to generalize to unseen variations in fabric defects. The images were manually annotated with defect type and bounding box information. The image annotation contains a class label, bounding box center (x,y), height, and width. For this research, datasets of the defects were collected from various garment manufacturers with their consent. However, Data collection is an ongoing process. Researchers interested in the dataset can contact us for access.
- Dataset
- WAYS OF USING GREENHOUSE GAS EMISSIONS FROM ANIMALS AND WASTE PRODUCTS IN AGRICULTURE WITH INNOVATIVE SOLUTIONSThe article is devoted to the questions about reducing negative effects of greenhouse gases on the atmosphere as a result of enteral fermentation in the intestines of animals into the environment and from the processes of cleaning, accumulation, and storage of manure from livestock premises. Analyzing legislation acts of countries, it has been discovered that their main purpose is to forecast and assess the total anthropogenic GHG emissions. In the republic, there is no instrumental method for measuring GHG emissions emitted by animals in a confined space. Multiplying the resulting total methane emissions by the coefficient of global warming potential, which is equal to 28%. Approximately 20,982.25 thousand tons of CO2-equivalent methane are released every year in this industry. This is 58% of the total emissions. Nitrous oxide emissions are 15239.72 thousand tons of CO2 equivalent. 2(42%). There was a total release of 319.547 million tons of CO2, 2.313 million tons of CH4, and 0.058 million tonsof N2O. N2 Research sgests that organic waste and GHGes released by confined animals can be converted into energy and organic fertilizer that is beneficial for the environment. Keywords: greenhouse gases, methane, carbon monoxide, bioreactor, solar collector
- Dataset
- Western Blot Raw Data for "A bioelectronic patch for organ-conformal, kirigami-structured electro-transfection towards precise intracellular delivery"
- Dataset
- dendrochemical data from PCCVCThis dataset contains concentrations of 15 trace elements in micro-grams per gram (µg/g), analyzed using ICP-MS in tree rings of Nothofagus pumilio, a tree species from the Andean region of Northern Patagonia. The analyzed elements include: Na, Mg, Al, K, Ca, Cr, Mn, Fe, Co, Ni, Cu, Zn, Se, Cd, and Ba. The data were obtained from tree ring samples of N. pumilio collected after two major volcanic eruptions of the Puyehue-Cordón Caulle Volcanic Complex (PCCVC) in 1921-22 and 2011. These results provide information on the concentrations of these elements in the trees' xylem, helping to understand the effects of volcanic eruptions and the biochemical processes that occur in trees after tephra fall. Data File: The concentration values for each element per sample, expressed in µg/g. Year: Year corresponding to the tree ring. Sapwood from 1916 to 1926; heartwood from 2005 to 2013. Location: Geographical location of the samples: 40°42'14" S, 71°57'31" W. Purpose of the data: These data can be used to study dendrochemical variations in Nothofagus pumilio trees, especially in response to volcanic eruptions, and to reconstruct past volcanic events based on the chemistry of tree rings. Expected Use: Researchers can use this data to assess how volcanic eruptions affect the absorption and distribution of trace elements in trees, providing new insights into the interaction between the environment and plants. These data can also be useful in studies of biogeography, climate change, and the biogeochemical cycling of elements in forest ecosystems.
- Dataset
- Revolutionizing Osteoporosis Diagnosis in Japan: A Low-Dose X-Ray Artificial Intelligence Model with Clinical Trial ValidationMetadata for research article 'Revolutionizing Osteoporosis Diagnosis in Japan: A Low-Dose X-Ray Artificial Intelligence Model with Clinical Trial Validation'
- Dataset
- Research Data for Paper: Fiber Deviation and Optimal Toolpath Strategies in Melt Electrowriting of Tubular ScaffoldsThis dataset contains stitched microscope image data of tubular scaffolds printed using melt-electrowriting (MEW). The images were used in the paper "Fiber Deviation and Optimal Toolpath Strategies in Melt Electrowriting of Tubular Scaffolds" by Neuhaus et al. to determine the deviation of the printed fiber from the programmed toolpath. Additionally, the G-code programs and the CNC encoder data from the printing process are included for reference.
- Dataset
- RPA in the Public Sector: A Systematic Literature ReviewRobotic Process Automation (RPA) is an emerging technology that has the potential to transform administrative management in the public sector by reducing workloads and optimizing resources. However, despite these benefits, the implementation of automation presents significant challenges, as experience indicates that the inappropriate selection of processes and the overestimation of their advantages often compromise the success of initiatives. The aim of this study is to conduct a systematic review of the literature to explore the state of the art on the use of RPA in the public sector, , identifying the key benefits, barriers and impact on staff performance. The search strategy used identified 318 studies, of which 20 were considered primary. The findings show that while automation can increase efficiency and reduce costs by automating repetitive tasks, successful implementation depends primarily on rigorous process selection and the digital readiness of the workforce.The organizational and technological challenges identified require strategic policies and a greater alignment between institutional needs and technological competencies. In addition, an analysis has been made of how the lessons learned in the private sector can be adapted to optimise the application of this technology in government contexts.
- Dataset
- 10.3389/fmolb.2024.1420691raw data of "A method for the analysis of the oligomerization profile of the Huntington's disease-associated, aggregation-prone mutant huntingtin protein by isopycnic ultracentrifugation" doi 10.3389/fmolb.2024.1420691
- Dataset
1
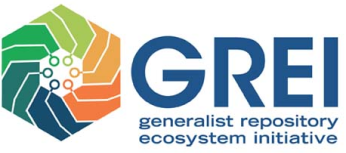
The Generalist Repository Ecosystem Initiative
Elsevier's Mendeley Data repository is a participating member of the National Institutes of Health (NIH) Office of Data Science Strategy (ODSS) GREI project. The GREI includes seven established generalist repositories funded by the NIH to work together to establish consistent metadata, develop use cases for data sharing, train and educate researchers on FAIR data and the importance of data sharing, and more.
Find out moreWhy use Mendeley Data?
Make your research data citable
Unique DOIs and easy-to-use citation tools make it easy to refer to your research data.
Share data privately or publicly
Securely share your data with colleagues and co-authors before publication.
Ensure long-term data storage
Your data is archived for as long as you need it by Data Archiving & Networked Services.
Keep access to all versions
Mendeley Data supports versioning, making longitudinal studies easier.
The Mendeley Data communal data repository is powered by Digital Commons Data.
Digital Commons Data provides everything that your institution will need to launch and maintain a successful Research Data Management program at scale.
Find out more