Search the repository
Recently published
- Data for Complexity at a Humid Interface: Throwing Light on Atmospheric CorrosionUploaded NAP-XPS, and VSFS data are described/discussed in a manuscript entitled 'Data for Complexity at a Humid Interface: Throwing Light on Atmospheric Corrosion', which is currently being considered for publication.
- Dataset
- LDHU3_18.1470Zinc finger - C3HC4 type (RING finger) containing protein; Leishmania donovani (HU3 strain)
- Dataset
- PIM treatment optimization with PM-TOM and Chat GPT 4A case study of the analysis and optimization of PIM treatment of an older adult. Analysis of ADRs caused by ADEs, DDIs, DCIs, DFIs, and the Beers risks in the admission and discharge treatments. Optimization of the discharge treatment to minimize the ADRs. ChatGPT review of the optimized treatments.
- Dataset
- LDHU3_18.1390Protein kinase A catalytic subunit | PKAC3; Leishmania donovani (HU3 strain)
- Dataset
- Raw data for "New mutations in the fission yeast spindle pole body protein Ppc89 lead to cell division defects" part 1Raw data associated with the manuscript within the title published in the Journal G3.
- Dataset
- The ATP-dependent DEAD-box RNA Helicase Dbp2 regulates the glucose/nitrogen stress response in baker’s yeast by modulating reversible nuclear retention and decay of SKS1 mRNAIn baker's yeast Saccharomyces cerevisiae, a small subset of total cellular mRNAs called special mRNAs are exported slowly and are retained preferentially in the nucleus. However, the mechanism of their slow export as well as the physiological significance of their nuclear retention remained elusive. In this work, we investigate the mechanistic aspect of the preferential nuclear retention of "Special" mRNAs in Saccharomyces cerevisiae, using SKS1 mRNA as a model special mRNA encoding a glucose-sensing serine/threonine kinase. Nuclear retention of the SKS1 mRNA triggered by a 202 nt “export-retarding” nuclear zip code (NZ) element promotes its rapid degradation in the nucleus by the nuclear exosome/CTEXT. We demonstrate that Dbp2p, an ATP-dependent DEAD-box RNA helicase binds to SKS1 and other special mRNAs and thereby inhibits their export by antagonizing with the binding of the export factors Mex67p/Yra1p. Consistent with this observation, a significant portion of these special mRNAs were found to localize into the cytoplasm in a yeast strain carrying a deletion in the DBP2 gene with the concomitant enhancement of its steady-state level and stability. This observation supports the view that Dbp2p promotes the nuclear retention of special mRNAs to trigger their subsequent nuclear degradation. Further analysis revealed that Dbp2p-dependent nuclear retention of SKS1 mRNA is reversible, which plays a crucial role in the adaptability and viability of the yeast cells in low concentrations of glucose/nitrogen in the growth medium. At high nutrient levels when the function of Sks1p is not necessary, SKS1 mRNA is retained in the nucleus and degraded. In contrast, during low glucose/nitrogen levels when Sks1p is vital to respond to such situations, the nuclear retention of SKS1 mRNA is relieved to permit its increased nuclear export and translation leading to a huge burst of cytoplasmic Sks1p.
- Dataset
- LuffaFolio: A Multifaceted Luffa Aegyptiaca Image DatasetThis dataset is divided into 3 parts; Each part consists of smooth luffa grading, diseases, and flowers respectively. These images were captured from different village fields of Faridpur, Bangladesh. To construct the dataset, we have considered Luffa Aegyptiaca commonly known as Smooth Luffa (Dhundal/ধুন্দল). This dataset consists of a total of 1,933 JPG images; the dimension of each image is 1728 x 1728 pixels. The total size of the dataset is 1.45 GB. The dataset contains 3 folders: Luffa_Diseases, Flowers, and Luffa_Grade. The detail of the dataset: • Luffa_Diseases: This dataset contains leaves of smooth luffa representing various diseases along with non-affected ones. The categories of this dataset are Alternaria Disease, Angular Spot Disease, Holed Leaves, Mosaic Virus, and Fresh Leaves. A total of 1,228 JPG raw images are presented in this folder. • Flowers: This dataset contains flowers of smooth luffa. There is only a single category of this dataset. A total of 362 JPG raw images are presented in this folder. These images represent various maturity stages of smooth luffa flowers. • Luffa_Grade: This dataset contains fresh and defective smooth luffa. The categories of this dataset are Fresh and Faulty Luffa. A total of 343 JPG raw images are presented in this folder. We have also uploaded a Resized version (224 X 224 pixels) of full dataset in zip format. --------------------------------------------------------------- Another version of this dataset is [Luffa Leaves Diseases] is given in zip format named: "Luffa_Diseases_REAL.zip" Train dataset contains 4000 images (800 original+augmented images each class) Train dataset contains 500 images (100 original and non-overlapped images each class)
- Dataset
- Plastic Waste Free DataKPI Calculation for Material Flow Analysis
- Dataset
- UTI IN RURAL NIGERIAN ADOLESCENTSThis is a data set for research on urinary tract infection among adolecents in rural, south east, Nigeria
- Dataset
- Raw data for "New mutations in the core Schizosaccharomyces pombe spindle pole body scaffold Ppc89 reveal separable functions in regulating cell division" part 6Raw data associated with the manuscript within the title published in the Journal G3.
- Dataset
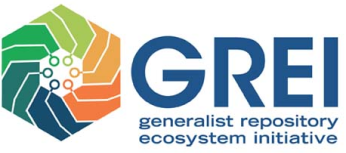
The Generalist Repository Ecosystem Initiative
Elsevier's Mendeley Data repository is a participating member of the National Institutes of Health (NIH) Office of Data Science Strategy (ODSS) GREI project. The GREI includes seven established generalist repositories funded by the NIH to work together to establish consistent metadata, develop use cases for data sharing, train and educate researchers on FAIR data and the importance of data sharing, and more.
Find out moreWhy use Mendeley Data?
The Mendeley Data communal data repository is powered by Digital Commons Data.
Digital Commons Data provides everything that your institution will need to launch and maintain a successful Research Data Management program at scale.
Find out moreData Monitor provides visibility on an institution's entire research data output by harvesting research data from 2000+ generalist and domain-specific repositories, including everything in Mendeley Data.
Find out more