Search the repository
Recently published
111981 results
- Genetic control of local mutation ratesCode relating to manuscript: Caballero M. and Koren, A. "Genetic control of local mutation rates". (2025) The code includes mutation rate bias and variability data, and the consensus LCL replication timing. Requests for further materials or data should be directed to the corresponding author.
- Dataset
- Exploring job satisfaction in fitness franchises: a study from a human talent perspectiveThis study examines job satisfaction in Mexican fitness franchises from a human talent perspective. The research is guided by the following key questions: What factors influence job satisfaction in fitness franchises? How do professional development opportunities and working conditions impact job satisfaction? What challenges or improvements can enhance overall job satisfaction and employee performance? Findings and Data Insights Data was gathered from six semi-structured interviews conducted in October 2023 with employees in different roles. The interviews explored work-life balance, motivation, professional development, relationships, and compensation. Internal communication emerged as the primary factor in job satisfaction. Poor communication from leadership led to uncertainty and dissatisfaction, whereas open dialogue and clear feedback boosted motivation. Interpersonal relationships also played a crucial role; strong team dynamics improved satisfaction, while unresolved conflicts and favouritism caused dissatisfaction. Work-life balance was another key element. Employees who managed professional and personal lives separately reported higher satisfaction. However, stress was a concern, especially for those handling heavy workloads or difficult customers. Flexible work arrangements and stress management strategies were suggested as solutions. Professional development was valued, but employees felt current training—mostly virtual—lacked engagement. Structured, in-person training could enhance skills and career progression. Compensation was generally viewed as fair, though some employees desired additional benefits like food vouchers and performance incentives. Small enhancements in benefits could positively impact morale. Data Collection and Interpretation Data was analysed using ATLAS.ti 23, applying a grounded theory approach to identify ten key themes: work-life balance, stress, communication, motivation, development, compensation, relationships, workplace environment, tasks, and overall satisfaction. Sentiment analysis showed neutral responses dominated, with a significant number reflecting negative emotions due to workplace challenges. The findings highlight the importance of targeted HR strategies. Recommendations include improving communication, fostering teamwork, offering structured training, and enhancing compensation. Aligning these with sustainable development goals can promote better working conditions and economic sustainability. By addressing these factors, fitness franchises in Mexico can improve retention, service quality, and organisational success. This study serves as a reference for future research and a strategic tool for HR management in similar industries.
- Dataset
- Raw Data Transparency: Photopolymerization Effects on Composite ResinsThe raw data presented in the attached files refer to the analyses conducted to evaluate the degree of conversion, flexural strength, elastic modulus, sorption, and solubility of three composite resins: Applic, Opallis, and Zirconfill. These resins were photopolymerized using three different devices: Bluephase, Valo, and Demi Plus. The data contained in these files represent the original measurements obtained during the experiments and were used to calculate the values presented in the study results. From this information, the necessary statistical analyses were performed to compare the performance of different composite resins under various photopolymerization conditions. It is important to highlight that the raw data provided have not undergone any filtering or additional processing beyond what was necessary for statistical analyses. Therefore, these values directly reflect the experimental conditions and may be useful for future reanalyses, validations, or comparative studies in the field of dental materials. The disclosure of these data reinforces the commitment to scientific transparency, allowing other researchers to access the primary information used to construct the conclusions of this study. This study aims to evaluate the impact of different photopolymerization devices (Bluephase, Valo, and Demi Plus) on the physico-mechanical properties of three composite resins (Applic, Opallis, and Zirconfill). The central hypothesis is that the choice of photopolymerization device directly influences the degree of conversion, flexural strength, elastic modulus, sorption, and solubility of the resins, thereby affecting their durability and clinical performance. Data Collection The data were obtained from laboratory experiments in which each composite resin was photopolymerized using the three mentioned devices. Measurements were then conducted to determine the following parameters: Degree of Conversion (GC%) – Measures the efficiency of monomer-to-polymer conversion during photopolymerization. Flexural Strength (MPa) – Evaluates the material’s ability to withstand applied forces before fracturing. Elastic Modulus (GPa) – Indicates the stiffness of the composite resin. Sorption and Solubility (µg/mm³) – Measures the material's ability to absorb liquids and dissolve in the oral environment.. The presented data can be interpreted by considering the influence of the photopolymerization devices on the physicomechanical properties of the composite resins. The interpretation is based on comparing the values obtained for each resin when subjected to the three devices (Bluephase, Valo, and Demi Plus), identifying patterns and trends.
- Dataset
- Empirical Analysis of Deep Neural Networks for Classifying and Identifying 316L and AZ31B Mg Metal Surface MorphologyDeep neural networks (DNNs) have demonstrated remarkable capabilities in image classification and pattern recognition, making them well-suited for material surface morphology analysis. This study presents an empirical analysis of DNNs for classifying and identifying surface morphology of 316L stainless steel and AZ31B magnesium alloy. High-resolution microscopic images of both metals were obtained and preprocessed to enhance feature extraction. Multiple DNN architectures, including convolutional neural networks (CNNs), were trained and evaluated to determine their efficacy in distinguishing surface textures influenced by processing methods such as machining, etching, and corrosion. Performance metrics such as accuracy, precision, recall, and F1-score were analyzed to assess model effectiveness. The results indicate that DNN models can successfully differentiate between the two metal surfaces with high accuracy, providing a robust framework for automated material characterization. These findings contribute to the advancement of intelligent material inspection systems, reducing manual effort and improving the reliability of surface morphology assessments in industrial applications.
- Dataset
- Metals and nutrients record in karstical aquifer
- Dataset
- The Notch inhibitor, FLI-06, increases the chemosensitivity of head and neck Squamous cell carcinoma cells to taxanes-based treatmentIn this study, we show that the use of novel, small-molecule inhibitors of Notch signaling, such as FLI-06, can have a beneficial effect on increasing the chemosensitivity of HNSCC to taxane-based chemotherapy. Inhibition of Notch signaling by FLI-06 alone virtually blocks the proliferation and growth of HNSCC cells in 2D cultures, which is accompanied by down-regulation of key Notch target genes and proteins. Mechanistically, FLI-06 treatment causes cell cycle arrest in the G1-phase and induction of apoptosis in HNSCC. Combining FLI-06 with Docetaxel shows a synergistic effect and partially blocks the cell growth of aggressive HNSCC cells via enhanced apoptosis and modification of c-JunS243 phosphorylation via GSK-3β inhibition. In conclusion, inhibition of Notch signaling in HNSCC cells that retain active Notch signaling significantly supports taxane-based anticancer activities via modulation of both the GSK-3β and the c-Jun. The dataset contains: - cell cycle raw data - PI/annexin raw data - qPCR raw data - Calculation and Visualization of synergy scores for Drug Combinations (SynergyFinder 3.0 software)
- Dataset
- COLOSS: Complex-scaled Optical and couLOmb Scattering SolverWe introduce COLOSS, a program designed to address the scattering problem using a bound-state technique known as complex scaling. In this method, the oscillatory boundary conditions of the wave function are transformed into exponentially decaying ones, accommodating the long-range Coulomb interaction. The program implements the general local optical potential and the Perey-Buck non-local optical potential, with all potential parameters included in a well-designed input format for ease of use. The design offers users direct access to compute S-matrices and cross-sections for scattering processes involving a projectile of any spin interacting with a spin-0 target. We provide thorough discussions on the precision of Lagrange functions and their benefits in evaluating matrix elements. Additionally, COLOSS incorporates two distinct rotation methods, making it adaptable to potentials without analytical expressions. Comparative results demonstrate that COLOSS achieves high accuracy when compared with the direct integration method, Numerov, underscoring its utility and effectiveness in scattering calculations.
- Dataset
- Exploring the strategic potential for Switzerland to reduce nitrogen and phosphorus surplus in agriculture.Calculation sheets to support the publication "Exploring the strategic potential for Switzerland to reduce nitrogen and phosphorus surplus in agriculture" published in Resources, Conservation & Recycling. The article explores the effect of a suite of food system interventions on nitrogen and phosphorus surplus in agriculture and is available freely from https://dx.doi.org/10.10.16/j.resconrec.2025.108239.
- Dataset
- Dataset on Shopping Mall Visits in Johannesburg, South Africahis dataset captures observations of consumer visits to three major shopping malls in Johannesburg, South Africa, from 2022 to 2023. The data, sourced from Fetch Analytics, utilizes smartphone signal tracking to provide insights into consumer behaviour. Key variables include mall name, visit frequency, distance travelled, and demographic indicators such as income and Living Standard Measure (LSM). The dataset allows for a granular analysis of how spatial and socio-economic factors influence shopping patterns in a fragmented retail landscape. This dataset is valuable for researchers investigating consumer behaviour, spatial economics, and urban retail planning.
- Dataset
- SUMO4 promotes SUMO deconjugation required for DNA double-strand break repair Figs and Supp Figs 5-6. Garvin et al.,SUMO4 promotes SUMO deconjugation required for DNA double-strand break repair Figs and Supp Figs 5-6. Garvin et al.,
- Dataset
1
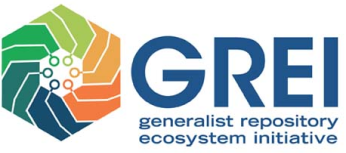
The Generalist Repository Ecosystem Initiative
Elsevier's Mendeley Data repository is a participating member of the National Institutes of Health (NIH) Office of Data Science Strategy (ODSS) GREI project. The GREI includes seven established generalist repositories funded by the NIH to work together to establish consistent metadata, develop use cases for data sharing, train and educate researchers on FAIR data and the importance of data sharing, and more.
Find out moreWhy use Mendeley Data?
Make your research data citable
Unique DOIs and easy-to-use citation tools make it easy to refer to your research data.
Share data privately or publicly
Securely share your data with colleagues and co-authors before publication.
Ensure long-term data storage
Your data is archived for as long as you need it by Data Archiving & Networked Services.
Keep access to all versions
Mendeley Data supports versioning, making longitudinal studies easier.
The Mendeley Data communal data repository is powered by Digital Commons Data.
Digital Commons Data provides everything that your institution will need to launch and maintain a successful Research Data Management program at scale.
Find out more