Search the repository
Recently published
119970 results
- Data from "Foraging Ants as Liquid Brains: Movement Heterogeneity Shapes Collective Efficiency"The data presented here were used to generate the figures and results of the research article titled "Foraging Ants as Liquid Brains: Movement Heterogeneity Shapes Collective Efficiency" (Fernández-López et al., 2025). To fully reproduce the analyses and figures, you will need to download the related code and instructions available in the GitHub repository cited below. The data are organized into two main folders: --- Raw Data --- The raw_data folder contains tracking data from food and no-food experiments, as well as simulations using the default parameter set (see Table S1 in the manuscript and the script parameters.py in the linked GitHub repository for details). Experimental data are stored as .csv files. Each file contains: *N-ind: identity of each individual *Frame: time in frames *Xmm, Ymm: x and y coordinates in millimeters *Crossings: whether an ant is interacting (1) or not (0) *Time_sec: time in seconds (Frame / 2) *node: the closest intersection in the Y-maze to the (x, y) position Simulation data are divided into two folders, each containing 100 files, one per realization of the model: The main folder, data, contains tracking data analogous to the experiments. These files are in Parquet format and should be read using libraries such as arrow (R) or pyarrow (Python). Each file includes: *T: time in seconds *Frame: time in frames (T x 2, as integer) *N: number of ants in the arena *pos: ant positions *food_target: whether ants are being directed to a food source (0 for no, 1 for the first food patch, 2 for the second food patch) The files in the food_data folder have the following columns: *node: location of the food in the lattice *t: time of detection by an ant *origin: currently unused (contains no meaningful data) --- Processed Data --- This folder contains RData files with pre-processed data for immediate use in reproducing analyses: *det.RData: data from food experiments *nf.RData: data from no-food experiments *hex.csv: Y-maze intersection coordinates (experiment) *hex_sims.csv: Y-maze intersection coordinates (simulation) Regarding simulations: rho_eps.RData contains a summary of results across the parameter space defined by "rho" (scout proportion) and "epsilon" (social copying proportion), as used in Figure 5 of the manuscript. simulations_n contains .csv files showing the number of ants in the arena (N) over time (Frame) for each simulation. Although this can be derived from the raw data, these files are included here to directly support Figure 4 reproduction.
- Phosphoru-2025In the present study, we conducted a field experiment with multiple levels of P addition to reveal the plant and soil traits and the associated P and C dynamics of L. chinensis, C. squarrosa, and S. grandis. In addition, we systematically examined the P and C dynamics throughout the plant–soil continuum. Phosphorus fertilization experiment began in 2014. The experimental area has been fenced since 2009. The initial soil Olsen-P concentration was 2.5 mg P kg-1, which is lower than the average in Inner Mongolia (http://www.imaaahs.ac.cn/). We designed five P addition treatments: 0, 1, 2.5, 5, and 12.5 g P m-2 year-1, using orthophosphate fertilizer (NaH2PO4).
- root morphological traits and KsThe data pertains to soil saturated hydraulic conductivity and root morphological traits, including:Soil saturated hydraulic conductivity (Ks),Root length density (RLD), Root surface area density (RSD), Root weight density (RWD) and Root volume density (RVD)
- Notes on replicating the results of “Melting fertility: The impact of extreme temperature on fertility intentions”Notes on replicating the results of “Melting fertility: The impact of extreme temperature on fertility intentions”
- Interphase formation of ethylenic polymers on photoreactive benzophenone-type adhesion promotersThe presented data supports the publication "Interphase formation of ethylenic polymers on photoreactive benzo-phenone-type adhesion promoters" and covers XPS data of polystyrene immobilized on silicon wafer surfaces modified by photoreactive adhesion promoters using UV light (365 nm LED). 220125 xps data table 1: This file contains XPS spectra on thick droplets of the adhesion promoters after synthesis to characterize their elemental stoichiometry and check the quality of the materials 230725 XPS data table S1: This file contains XPS data of silicon wafers after modified with adhesion promoter PS-K after immobilization of polystyrene (290 kDa Mp) using increasing irradiation doses of UV light (2-1800 s). 250704 XPS data table S2: This file contains XPS data of licon wafers after modified with adhesion promoter triPS-F after immobilization of polystyrene of increasing molecular weight (3.3-5150 kDa Mp) at a single (maximum) UV irradiation dose corresponding to 1800 s. The file also contains data on 2 other immobilized polymer films using the same conditions but PMMA and poly alpha-methylstyrene (PaMS). The data is provided in form of Vamas-files, which can be read by any XPS data analysis program.
- Phylogenomics untangles the metabolic potential of Picochlorum tauri, a new picoalgal species causing a winter bloom in the Mediterranean Thau lagoon.Data repository for Picochlorum tauri , a new picoalgal species causing a winter bloom in the Mediterranean Thau lagoon. In this repository, genome sequences and annotation data can be found. Additionally, comparative genomics analysis results of the interested species with other Trebouxiophyceae are included.
- Movement_VigorMain R code (GLMM analyses) and data for the project: "Altruistic punishment in action: movement vigor in neuroeconomic choice"
- LINF_220014500Protein of unknown function - conserved; Leishmania infantum (strain JPCM5)
- SWET model data for quantifying the trade-offs in global energy transitionsThis dataset contains a model for planning energy transitions that looks at the extra energy available for optional use to meet social development goals, after considering the energy costs related to building and running the energy system, along with the energy needed to support ecosystems. The model considers four significant parameters to ascertain the rising energy costs associated with the decarbonization process. • Diminishing EROI trends for both fossil fuels and renewable energy sources • The pace of establishing renewable energy infrastructure • Ecosystem Maintenance Energy (ESME) • The energy expenditure associated with carbon sequestration This dataset is cited in the paper "Quantifying the Trade-offs in Global Energy Transitions: How Economic Growth Undermines Carbon Targets and Sustainable Surplus Energy," published in the journal Renewable and Sustainable Energy Reviews. https://doi.org/10.1016/j.rser.2025.116027
- Modelling the Impact of Bikeability Cycle Training on the Number of People Killed or Seriously Injured on UK RoadsRaw data, statistical analyses outputs, and the associated TRL report, from the paper entitled 'Modelling the Impact of Bikeability Cycle Training on the Number of People Killed or Seriously Injured on UK Roads'
1
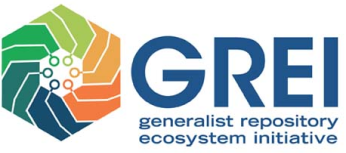
The Generalist Repository Ecosystem Initiative
Elsevier's Mendeley Data repository is a participating member of the National Institutes of Health (NIH) Office of Data Science Strategy (ODSS) GREI project. The GREI includes seven established generalist repositories funded by the NIH to work together to establish consistent metadata, develop use cases for data sharing, train and educate researchers on FAIR data and the importance of data sharing, and more.
Find out moreWhy use Mendeley Data?
Make your research data citable
Unique DOIs and easy-to-use citation tools make it easy to refer to your research data.
Share data privately or publicly
Securely share your data with colleagues and co-authors before publication.
Ensure long-term data storage
Your data is archived for as long as you need it by Data Archiving & Networked Services.
Keep access to all versions
Mendeley Data supports versioning, making longitudinal studies easier.
The Mendeley Data communal data repository is powered by Digital Commons Data.
Digital Commons Data provides everything that your institution will need to launch and maintain a successful Research Data Management program at scale.
Find out more